Apocalypse or ally? Managing AI to advance sustainability
Capturing the benefits and minimizing the risks of artificial intelligence will require practical measures that build confidence and trust. Read More
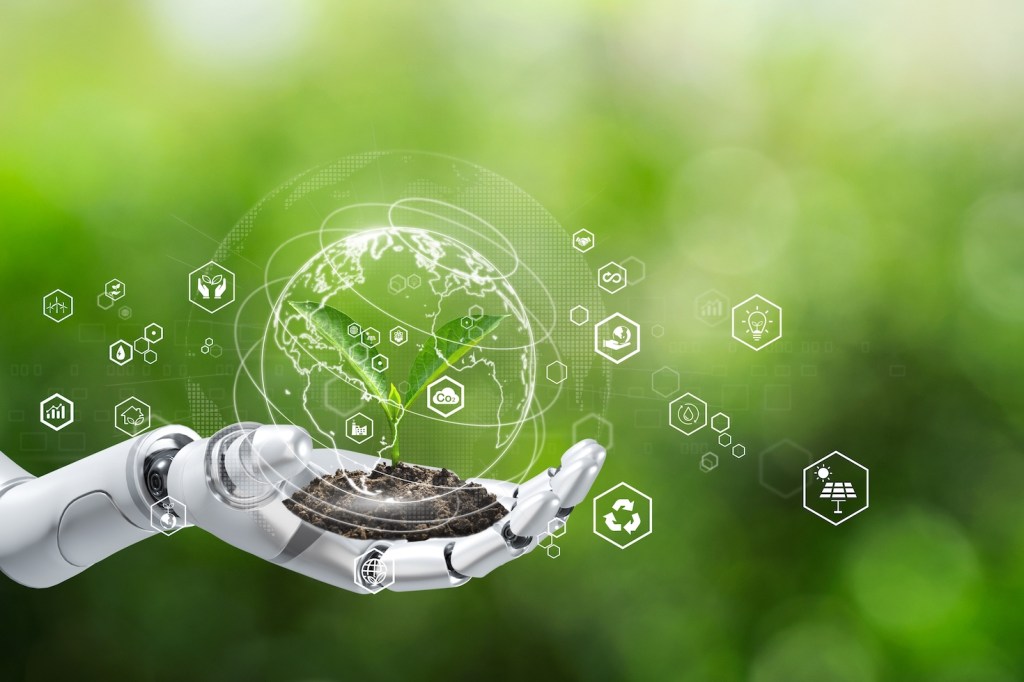
Image via Shutterstock/chayanuphol
By now, the critics and proponents of artificial intelligence (AI) have our attention. For the past several months there has been a steady downpour of analyses and claims that range from AI presenting a “risk of extinction … alongside other societal scale risks such as pandemics and nuclear war” to finding miraculous medical breakthroughs through searches of “genetic haystacks.”
One thing is for sure: AI has not suddenly appeared, even though the behavior of hedge funds and other investors and the financial press might cause you to think otherwise. Like most major technological innovations, AI has been on an evolutionary path for some time.
Similar to its growing impacts on the economy and other aspects of social life, AI will stimulate a rethinking of its relationship to sustainability. AI will confer a series of societal benefits, while also embedding the possibility of major disruptions and risks.
What sustainability benefits can we expect from AI?
There are several major benefit categories from investing in and applying AI technologies. They include:
- Unifying public health and environmental data. The continuing degradation of biodiversity and related aquatic and terrestrial ecosystems from human activities has yielded an outcome in which human health can no longer be sufficiently protected, as environmental support systems necessary for human life — air, land and water — continue to deteriorate. The promise of AI and related digital technologies lies in the fact that both nature and human infrastructure are increasingly rich sources of data, and effectively designed data-based algorithms can enable decision-makers at all levels to detect changes in viability and status at both specific sites (ecosystems, cities) and at the system level. These insights can create new opportunities for problem prevention and remediation.
- Building new supply chain business models. Individual companies generate complicated supply chains, which create massive structural barriers to the design of information reporting systems, timely access to data and alignment of goals and metrics. On a more basic level, many customers have no idea who their lower-tier suppliers are. As companies cope with the newer economic realities of geopolitical risks in the Asia-Pacific region, post-pandemic near-shoring of supply chains and accelerating climate change risks, they are imagining new business models for supply chain management. A critical component of this new thinking is investment in digital data systems, including enhanced AI with more common data reporting platforms arrayed around more consistent goals and metrics. Practical applications of such enhanced supply chain AI include analytics that optimize energy efficiency, water conservation, air quality and safety performance in factories, warehouses, distribution centers and ships. An integrated data-driven supply chain business model would enable electronic communication among suppliers and customers and achieve significant cost savings and equally important operational efficiencies.
- Realizing open innovation opportunities. Pollution from the continuing increase in plastics production (9 billion tons to date, with a projection of 11 billion tons by 2025) is detected in soils, crops and on the ocean floor. There is growing scientific evidence that microplastics are being transported long distances in the air where they can be absorbed in the human lung or alter cloud formation and composition, thus potentially changing temperature and rainfall patterns. The scale of the research challenge to develop more definitive data on these negative effects dwarfs the capability of any single research institution, government agency or industry sector. An open innovation research strategy can be developed to transcend traditional research planning, but it would require both funders in government, business and foundations and stakeholders to abandon their traditional silos and organize their efforts to create data that is universally owned and publicly transparent. Protocols for AI research and content development are especially important in designing microplastics research and modeling for global scale to better account for the dispersion, concentration and impacts of microplastics in the environment.
Major sustainability-related AI risks
While seeking to capture the benefits of AI technologies, it is critically important to be mindful of their risks. Some principal AI risks include:
- Inserting false data sets to misinform regulators, investors, consumers and other stakeholders. Today, there are numerous debates over which data is the most important for evaluating risks to environment, social and governance (ESG), communicating the sustainability benefits of consumer products, and verifying national emissions estimates to comply with international treaties. The opportunities for generating fraudulent AI content in these and other applications are significant and will require additional data management controls to be instituted.
- Worsening inequality, diversity and inclusion. Results of many studies to date conclude that facial recognition technologies consistently underrepresent, misidentify and/or distort features of non-white populations. Other social surveys frequently undercount members of racial minorities. These and other flaws in current methodologies and technologies generate a number of negative consequences ranging from challenges faced by individual passengers in boarding airplanes, access to credit and opportunities for employment. A root cause in these flaws lies in how researchers and their business sponsors often design projects to optimize their perception of existing human managed processes that are unrepresentative of population diversity. This ultimately leads to discrimination, more automated substitutes for human labor and a loss of jobs.
- Disrupting social behavior. Up to this juncture, analyses of AI impacts have focused principally on the ability to focus user attention as measured by clicks, participation in online clubs, purchase of goods and influence upon political behavior. Israeli historian and philosopher Yuval Noah Harari warns that the new generation of AI will transform the battlefront “from attention to intimacy.” Because of AI’s growing mastery of language, it could even “form intimate relationships with people, and use the power of intimacy to change our opinions and worldviews” on topics as varied as our political disposition, view of culture and history, and food, sex and religious preferences. Opponents of the transition away from internal combustion engines, connection of renewable energy production to the electricity grid and use of evidence-based risk assessments, to name a few, have a growing number of AI-designed weapons at their disposal to confuse the public and disrupt decision-making by governments and businesses.
Some proposed rules of the road
How can we see through the AI fog and extract what we need to make sensible decisions that advance sustainability? Some practical measures that build confidence and trust among multiple AI developers and consumers are a logical place to start. They include:
- Practicing more aggressive transparency. Making decisions more sustainable depends upon access to accurate and verifiable information. Given the rapid evolution of AI technologies, those developing new algorithms to guide AI applications should more explicitly present their methodologies, identify the data sets they are collecting and analyzing and declare the key assumptions and values to mimic or substitute the human behavior they are introducing.
- Developing AI data standards and certifications. This effort can coexist with and support more effective AI oversight at multiple levels. Individual industry sectors can prepare voluntary standards governing the development and use of AI technologies, regulatory bodies in the U.S., EU and beyond can develop and enforce minimum standards, and international standard setting organizations should define best management practices and optimize certification processes.
- Expanding multi-stakeholder governance processes.. Neither government agencies nor the private sector can effectively manage AI-related risks. Government is too slow and, at times, too politicized to keep pace with the rapidly evolving suite of AI technologies. The private sector has historically been unsuccessful in balancing profitability with the protection of the public interest and planet. More hybrid examples of governance — such as the recently launched Global Energy Alliance for People and Planet, or the satellite methane data collection program managed by the Environmental Defense Fund to improve the accountability of fossil fuel producers for their emissions — show how major institutions can share authority and accountability in the service of specific objectives. Similar opportunities await the further evolution of AI technologies.
Companies and governments are rapidly investing in digital data technologies, including AI. The sustainability community, already in catch-up mode, finds itself at a critical moment of reckoning for how best to adapt to a new technology era that, for good or for ill, can potentially transform both our planet and ourselves.
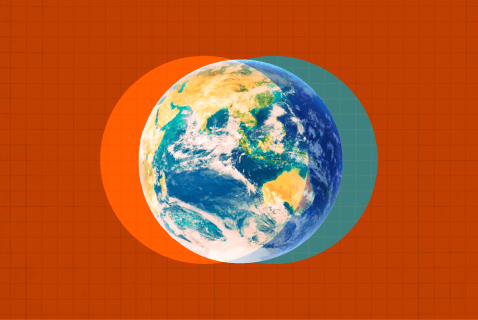